Índices de sentimiento regionales y su asociación con indicadores oportunos de actividad económica en México, 2016-2021
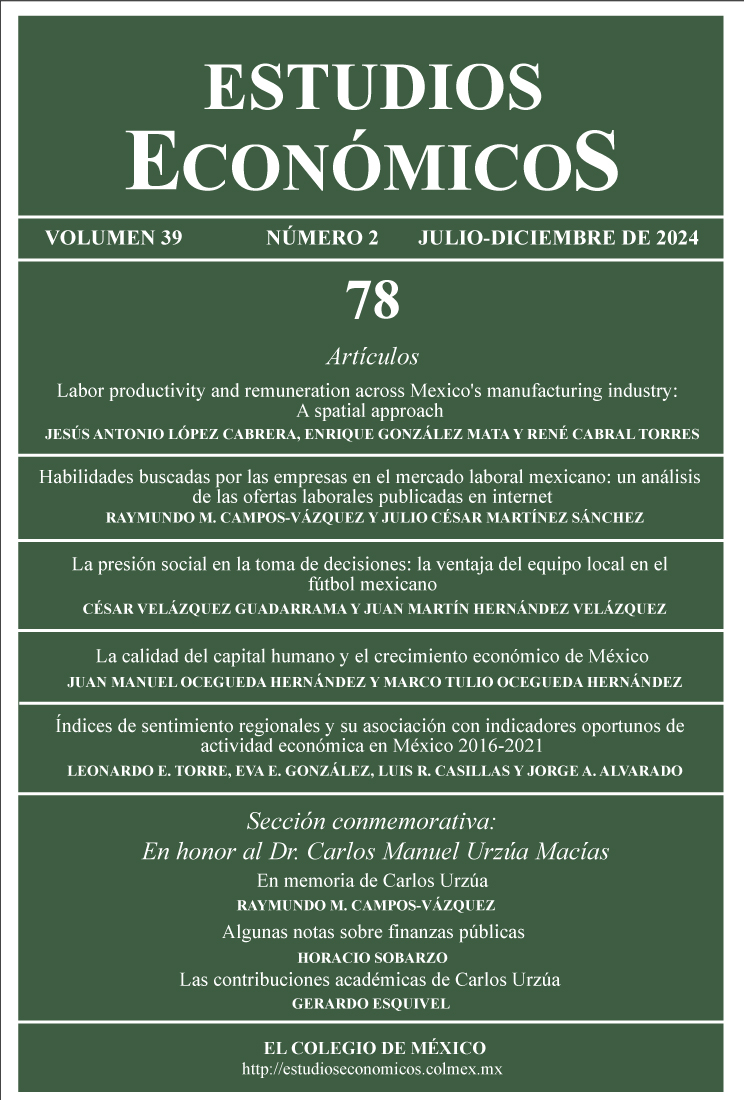
Publicado 2024-07-31
Palabras clave
- análisis de sentimientos,
- aprendizaje automático,
- análisis regional,
- México
Cómo citar
Resumen
Estimamos índices de sentimiento a nivel regional y nacional utilizando información en formato de texto del Programa Trimestral de Entrevistas a Directivos, empleada para la elaboración del Reporte sobre las Economías Regionales del Banco de México, referente a los factores que los entrevistados consideran que afectaron, afectan o pudieran afectar la actividad económica en su sector o entidad federativa. Estos índices, estimados con información de los programas de entrevistas trimestrales llevados a cabo entre enero de 2016 y enero de 2021, son posteriormente asociados con diferentes indicadores de actividad económica regional y nacional publicados por el INEGI, obteniéndose correlaciones positivas y estadísticamente significativas entre los índices de sentimiento y algunos indicadores de actividad económica. Dado que estos índices de sentimiento pueden obtenerse con mayor rapidez que la mayoría de los indicadores tradicionales de actividad económica aquí analizados, el trabajo destaca la relevancia de la información en formato de texto contenida en el Programa Trimestral de Entrevistas a Directivos para complementar la obtenida con indicadores tradicionales.
Descargas
Citas
- Algaba, A., D. Gardia, K. Bluteau, S. Borms y K. Boudt. 2020. Econometrics meets sentiment: An overview of methodology and applications, Journal of Economic Surveys, 34(3): 512-547. DOI: https://doi.org/10.1111/joes.12370
- Alloghani, M., D. Al-Jumeily, J. Mustafina, A. Hussain y A.J. Aljaaf. 2020. A systematic review on supervised and unsupervised machine learning algorithms for data science, en M. Berry, A. Mohamed y B. Yap (eds.), Supervised and Unsupervised Learning for Data Science, Springer. DOI: https://doi.org/10.1007/978-3-030-22475-2_1
- Anandarajan, M., C. Hill y T. Nolan. 2019. Semantic space representation and latent semantic analysis, en Practical Text Analytics, Advances in Analytics and Data Science, Vol. 2, Springer. DOI: https://doi.org/10.1007/978-3-319-95663-3
- Aragón, M., M. Carmona, M. Montes, H. Escalante, L. Villaseñor y D. Moctezuma. 2019. Overview of MEX-A3T at IberLEF 2019: Authorship and aggressiveness analysis in Mexican Spanish tweets, documento presentado en SEPLN Workshop on Iberian Languages Evaluation Forum (IberLEF), Bilbao.
- Arora, S. 2021. Data mining vs. machine learning: The key difference, www.simplilearn.com/data-mining-vs-machine-learning-article.
- Athey, S. 2018. The impact of machine learning on Economics, en A. Agrawal, J. Gans y A. Goldfarb (eds.), The Economics of Artificial Intelligence: An Agenda, National Bureau of Economic Research.
- Azqueta-Gavaldón, A., D. Hirschbühl, L. Onorante y L. Saiz. 2020. Economic policy uncertainty in the Euro area: An unsupervised machine learning approach, Working Paper Series No. 2359, European Central Bank. DOI: https://doi.org/10.2139/ssrn.3516756
- Banco de México. 2015. Informe trimestral octubre-diciembre, https://www.banxico.org.mx/publicaciones-y-prensa/informes-trimestrales/%7B94CE88E5-3F13-4707-8038-30A0F49D6E47%7D.pdf.
- Banco de México. 2016a. Informe trimestral enero-marzo, https://www.banxico.org.mx/publicaciones-y-prensa/informes-trimestrales/%7BA3AA2471-B70C-DAA2-01DF-EA06C6546B6A%7D.pdf
- Banco de México. 2016b. Informe trimestral abril-junio, https://www.banxico.org.mx/publicaciones-y-prensa/informes-trimestrales/%7BAD156BB0-60B7-947E-A9EF-9C96AB882667%7D.pdf.
- Banco de México. 2016c. Informe trimestral julio-septiembre, https://www.banxico.org.mx/publicaciones-y-prensa/informes-trimestrales/%7BD093DF85-0D83-3DD2-A533-3431AFAFE3A1%7D.pdf.
- Banco de México. 2019. Informe trimestral octubre-diciembre, https://www.banxico.org.mx/publicaciones-y-prensa/informes-trimestrales/%7B0DED33B2-FF70-345D-53BE-77EA35A0D743%7D.pdf.
- Banco de México. 2020a. Informe trimestral enero-marzo, https://www.banxico.org.mx/publicaciones-y-prensa/informes-trimestrales/%7B23C2DCA8-4AD3-FBE0-B0BF-4D30C8066B84%7D.pdf.
- Banco de México. 2020b. Reporte sobre las economías regionales julio-septiembre, https://www.banxico.org.mx/publicaciones-y-prensa/reportes-sobre-las-economias-regionales/%7B8427BCB2-D8F2-C28A-8DD4-EB8DD9770681%7D.pdf.
- Banco de México. 2020c. Informe trimestral octubre-diciembre, https://www.banxico.org.mx/publicaciones-y-prensa/informes-trimestrales/%7B81BD569D-DD6E-885A-A67F-5664A37B4148%7D.pdf.
- Banco de México. 2021. Informe trimestral enero-marzo, https://www.banxico.org.mx/publicaciones-y-prensa/informes-trimestrales/%7B49D9C039-CE93-FC5A-59A6-DFF7579FDB26%7D.pdf.
- Barocas, S. 2014. Data mining and the discourse on discrimination, documento presentado en KDD '23: Proceedings of the 29th ACM SIGKDD Conference on Knowledge Discovery and Data Mining, California.
- Benhabib, J. y M. Spiegel. 2017. Sentiment and economic activity: Evidence from U.S. states, NBER Working Paper No. 23899. DOI: https://doi.org/10.3386/w23899
- Blei, D. 2011. Introduction to probabilistic topic models, Communications of the ACM, 55(4): 77-84. DOI: https://doi.org/10.1145/2133806.2133826
- Bojanowski, P., E. Grave, A. Joulin y T. Mikolov. 2017. Enriching word vectors with subword information, Transactions of the Association for Computational Linguistics, 5: 135-146. DOI: https://doi.org/10.1162/tacl_a_00051
- Boyd, D. y K. Crawford. 2012. Critical questions for big data: Provocations for a cultural, technological, and scholarly phenomenon, Information, Communication and Society, 15(5): 662-679. DOI: https://doi.org/10.1080/1369118X.2012.678878
- Campos-Vázquez, R.M., B. López-Araiza y E. Sergio. 2020. Grandes datos, Google y desempleo, Estudios Económicos, 35(1): 125-151. DOI: https://doi.org/10.24201/ee.v35i1.399
- Cho, K., B. Van Merriënboer, C. Gulcehre, D. Bahdanau, F. Bougares, H. Schwenk y Y. Bengio. 2014. Learning phrase representations using RNN encoder-decoder for statistical machine translation, ArXiv preprint, arXiv:1406.1078. DOI: https://doi.org/10.3115/v1/D14-1179
- D’Andrea, A., F. Ferri, P. Grifoni y T. Guzzo. 2015. Approaches, tools and applications for sentiment analysis implementation, International Journal of Computer Applications, 125(3): 26-33. DOI: https://doi.org/10.5120/ijca2015905866
- De Bondt, G. y S. Schiaffi. 2015. Confidence matters for current economic growth: Empirical evidence for the Euro-area and the United States, Social Science Quarterly, 96(4): 1027-1040. DOI: https://doi.org/10.1111/ssqu.12181
- Devlin, J., M. Chang, K. Lee y K. Toutanova. 2018. BERT: Pre-training of deep bidirectional transformers for language understanding, ArXiv preprint, arXiv: 1810.04805.
- Díaz, M. y J. Huerta. 2020. Co-movimiento entre los índices de confianza del consumidor de México y Estados Unidos 2001-2018, Economía, Sociedad y Territorio, 20(62): 123-150. DOI: https://doi.org/10.22136/est20201379
- Doerr, S., L. Gambacorta y J. Serena. 2021. Big data and machine learning in central banking, BIS Working Paper No. 930, Bank for International Settlements.
- Garrett, T., R. Hernández-Murillo y M. Owyang. 2004. Does consumer sentiment predict regional consumption?, Federal Reserve Bank of St. Louis Review, 87(2): 123-35. DOI: https://doi.org/10.20955/r.87.123-135
- Gentzkow, M., K. Bryan y M. Taddy. 2019. Text as data, Journal of Economic Literature, 57(3): 535-574. DOI: https://doi.org/10.1257/jel.20181020
- González, C. y M. Herman. 2020. Foreign exchange forecasting via machine learning, https://cs229.stanford.edu/proj2018/poster/76.pdf.
- Hatzivassiloglou, V. y K. McKeown. 2002. Predicting the semantic orientation of adjectives, en 35th Annual Meeting of the Association for Computational Linguistics, Madrid, Association for Computational Linguistics.
- Jurafsky, D. y J. Martin. 2020. Speech and language processing, tercera edición (borrador), https://web.stanford.edu/~jurafsky/slp3/.
- Kay, M, C. Matuszek y S. Munson. 2015. Unequal representation and gender stereotypes in image search results for occupations, en Proceedings of the 33rd Annual ACM Conference on Human Factors in Computing Systems, Nueva York, Association for Computational Linguistics. DOI: https://doi.org/10.1145/2702123.2702520
- Kim, S. y E. Hovy. 2004. Determining the sentiment of opinions, en Proceedings of International Conference on Computational Linguistics, Ginebra, Coling. DOI: https://doi.org/10.3115/1220355.1220555
- Korab, P. 2021. Use of machine learning in economic research: What the literature tells us, https://towardsdatascience.com/use-of-machine-learning-in-economic-research.
- Medhat, W., A. Hassan y H. Korashy. 2014. Sentiment analysis algorithms and applications: A survey, Ain Shams Engineering Journal, 5(4): 1093-1113. DOI: https://doi.org/10.1016/j.asej.2014.04.011
- Miller, C. y C. Dwork. 2015. Algorithms and bias: Q. and A., New York Times,
- http://www.nytimes.com/2015/08/11/upshot/algorithms-and-bias-q-and-a-with-cynthia-dwork.html.
- Moritz, H. 2014. How big data is unfair, https://medium.com/@mrtz/how-big-data-is-unfair-9aa544d739de.
- Muñiz, V. 2020. Una consulta sobre técnicas de aprendizaje automático, Entrevista presencial, Centro de Investigación en Matemáticas A.C. Monterrey.
- Nasukawa, T. y J. Yi. 2003. Sentiment Analysis: Capturing Favorability using Natural Language Processing, Nueva York, Association for Computing Machinery. DOI: https://doi.org/10.1145/945645.945658
- Pang, B., L. Lee y S. Vaithyanathan. 2002. Thumbs up? Sentiment classification using machine learning techniques, en Proceedings of the 2002 Conference on Empirical Methods in Natural Language Processing (EMNLP 2002), Nueva York, Association for Computational Linguistics.
- Pinto, S. 2019. Sentiment analysis of the fifth district manufacturing and service surveys, Economic Quarterly, 105(9): 133-164. DOI: https://doi.org/10.21144/eq1050301
- Prabowo, R. y M. Thelwall. 2009. Sentiment analysis: A combined approach, Journal of Informetrics, 3(2): 143-157. DOI: https://doi.org/10.1016/j.joi.2009.01.003
- Rho, C., R. Fernández y B. Palma. 2021. A sentiment based indicator for the Mexican financial sector, Documento de Investigación No. 2021-04, Banco de México. DOI: https://doi.org/10.36095/banxico/di.2021.04
- Rosenbaum, P. y D. Rubin. 1983. The central role of the propensity score in observational studies for causal effects, Biometrika, 70(1): 41-55. DOI: https://doi.org/10.1093/biomet/70.1.41
- Salhin, A., M. Sherif y E. Jones. 2016. Managerial sentiment, consumer confidence and sector returns, International Review of Financial Analysis, 47: 24-38. DOI: https://doi.org/10.1016/j.irfa.2016.06.009
- Santero, T. y N. Westerlund. 1996. Confidence indicators and their relationship with changes in economic activity, OECD Working Papers No. 170.
- Stsiopkina, M. 2022. Hard vs. soft data: The difference, https://oxylabs.io/blog/hard-data-vs-soft-data.
- Suss, J. y H. Treitel. 2019. Predicting bank distress in the UK with machine learning, Staff Working Paper No. 831, Bank of England. DOI: https://doi.org/10.2139/ssrn.3465753
- Sutton, R. y A. Barto. 2018. Reinforcement Learning, Estados Unidos, The MIT Press.
- Sweeney, L. 2013. Discrimination in on-line ad delivery, Queue, 11(3): 1-20. DOI: https://doi.org/10.1145/2460276.2460278
- Taboada, M., J. Brooke, T. Tofiloski, K. Voll y M. Stede. 2011. Lexicon-based methods for sentiment analysis, Computational Linguistics, 37(2): 267-307. DOI: https://doi.org/10.1162/COLI_a_00049
- Turney, P. 2002. Thumbs up or thumbs down? Semantic orientation applied to unsupervised classification of reviews, ArXiv preprint, arXiv:cs/0212032. DOI: https://doi.org/10.3115/1073083.1073153
- Uno, Y. y K. Adachi. 2019. Calculating non-response bias in firms’ inflation expectations using machine learning techniques, Working Paper Series No.19-E-17, Bank of Japan.
- Wallach, H. 2014. Big data, machine learning, and the social sciences: Fairness, accountability, and transparency, https://medium.com/@hannawallach/big-data-machine-learning-and-the-social-sciences-927a8e20460d.
- Weng, L. 2021. Learning with not enough data. Part 1: Semi-supervised learning, https://lilianweng.github.io/posts/2021-12-05-semi-supervised/
- Wiesalla, L. 2021. The machine learning workflow. Concepts and applications, https://www.nextlytics.com/blog/machine-learning-workflow.
- Witten, I., E. Frank, M. Hall y C. Pall. 2017. Data Mining: Practical Machine Learning Tools and Techniques, Elsevier, Morgan Kaufmann Publishers.